Geospatial Modeling Using Netlogo
Is it possible to use two maps in a netlogo model? If yes, how to combine or to import it to netlogo?The first map has land use value and the second map has the land price value. Actually I am still studying it, but as far as I know that we can import map to netlogo that its format.asc, txt or.shp. Asc and txt format are also known as raster map. I have imported a raster map to my model.
1) Pattern Oriented Modeling looks at time to find answers about the subject being studied. In contrast, CA modeling techniques are concerned with quant. Many of the models where created using NetLogo. I would like to thank the Students of CSS 645: Spatial Agent-based Models of Human-Environment Interactions for their participation in the class. Posted by Andrew Crooks at.
I want to import one more raster map to the model since I need some values from the map to support the model but so far I still can't do it. It seems that netlogo model can only use one raster map. But, for shp format map or shapefile we can import more than one map to the model. If it's true that netlogo only able to import 1 raster map than I hv to use the shp map to substitute it.–Aug 29 '15 at 14:50.
. 3k Downloads.AbstractThis paper presents an extension to the agent-based model “Creative Industries Development–Urban Spatial Structure Transformation” by incorporating GIS data. Three agent classes, creative firms, creative workers and urban government, are considered in the model, and the spatial environment represents a set of GIS data layers (i.e.

Road network, key housing areas, land use). With the goal to facilitate urban policy makers to draw up policies locally and optimise the land use assignment in order to support the development of creative industries, the improved model exhibited its capacity to assist the policy makers conducting experiments and simulating different policy scenarios to see the corresponding dynamics of the spatial distributions of creative firms and creative workers across time within a city/district. The spatiotemporal graphs and maps record the simulation results and can be used as a reference by the policy makers to adjust land use plans adaptively at different stages of the creative industries’ development process. The last two decades saw the emergence of creative industries and globally an interest in cultivating them to promote economic growth (Cunningham ). The underlying rationale is that the creative industries can generate new businesses, especially in urban areas (Flew; Howkins ), and, by doing so, increase the employment rate (Higgs et al.; Mossig ). Furthermore, the potential of creative industries for urban regeneration (Evans; Pratt ), urban innovation (Scott; Stam et al. ) and urban sustainable development (Kakiuchi ) has been highlighted by multiple authors.
There are discordant voices from sceptics (Flew; Flew and Cunningham ), and comments that the term “creative industries” itself lacks rigour (Garnham; Pratt ). Notwithstanding, wide debates indicate a direct linkage between these industries and economic development.These streams of research, however, have overlooked the spatiality and location preferences of creative industries. For urban policy making and urban land use governance, understanding of these location preferences and their influence on urban land use can help urban planners and governments to formulate customised plans and policies.
One conclusion regarding the location preferences of creative firms is that they are primarily determined by “soft” factors, such as creative milieu, cultural diversity and knowledge spillovers (Bathelt et al.; Florida ). However, there is evidence implying that location preferences are more complex, as they are influenced by factors such as personal social networks (Musterd and Murie ) and environmental amenities, in addition to traditional “hard” factors such as cultural facilities, infrastructures, public transport services and shopping centres (Dainov and Sauka ). The combination of these factors presents new challenges to the traditional location analysis based on economics-inspired mathematical models.Urban planning involves complex negotiations and is characterised by evolutionary change (de-Roo; Silva ). It is therefore important to include other dimensions into the study of creative industries. One way to expand traditional research is to examine the dynamics by applying cluster theory (Keane ).
This approach helps to explain the emergence of creative industrial parks or spatial concentrations of creative industries; however, the analytical focus is limited to internal interactions among firms within clusters and their connection with innovation (Chapain et al. ) or urban governance (Keane ). Nevertheless, in this approach, the dynamics of urban land use change that result from creative industries’ development, still, are not well explored. Although this aspect has been touched in urban regeneration analysis (Montgomery,; Pratt ), a crucial element relating to the pattern in the numbers of the creative industries and the availability of the creative workforce have not been part of the scope of research. That is, the interdependence of firms and workers has been ignored.
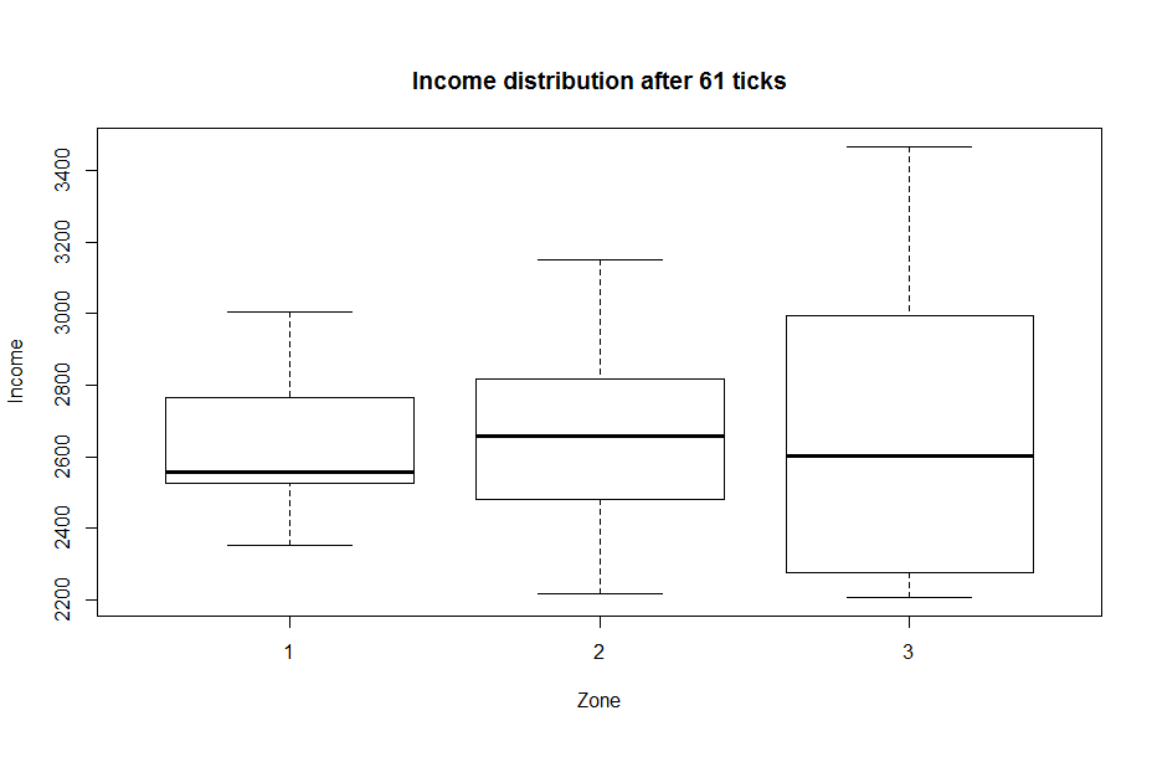
Furthermore, the temporal dimension of the spatiality of the dynamics has been overlooked, which limits the applicability of the existing research to urban planning practice—in particular to urban land use planning.Efforts to study the complexity of urban land use change by means of “soft Artificial Intelligence” have been made since the 1960s. However, it was not until the 1990s that these efforts began to thrive (Batty et al.; Wu and Silva ). A result of these computer models has been a shift from strongly deterministic ways of representing urban systems to more complex adaptive forms that more easily adjust to actual conditions and include more stochastic and heterogeneous characteristics, when dealing with urban land use dynamics (Silva and Wu ). Serial para nero platinum 2019.
However, few simulation models of internal urban land changes that could result the development of creative industries exist. An example is the CID-USST (Creative Industries Development–Urban Spatial Structure Transformation) model developed by Liu and Silva (, ) in NetLogo, a free agent-based modelling platform credited to Wilensky ( ). This model was later enhanced by Wang et al. ( ).In early applications of CID-USST model, the environment was very simply an abstraction of the study area, Nanjing city, China. The study area was represented by a homogenous space with one urban centre and a concentric division of the urban space (Liu and Silva ). This simplified spatial representation limited the applicability of the model to other cases, given that most large cities are multi-centred and not concentrically organised, but rather cover irregular geographical areas and display heterogeneous socio-economic characteristics.
In addition, the abstract space in the original model reduced its usefulness in planning practice as it was impossible to project the activities and changes occurring in the model at the urban land level at which planning takes place. To expand the capabilities of the CID-USST model, it becomes necessary to adjust the dataset structure and the algorithm. First, it is necessary to relax the monocentric assumption. Second, as the factors determining the location preferences of the firms and the workers vary across cases (Dainov and Sauka; Žaucer et al. ), the new model must be able to process a broader array of factors beyond the eight factors for firms and the five factors for workers in the original formulation of the model details can be seen in Liu and Silva ( ). Third, to facilitate urban policy making, the new model needs to enable the model users to customise policy combinations by referring to local context. Fourth, abstract urban space needs to be replaced by real city maps so that locations/sites where activities happen and are of interest to the urban government can be accurately indentified (Table ).
AspectCIS-USST modelCID-USST-GIS modelSpatial environmentConcentric city/homogeneous data of the case study (“idealised” city of Nanjing)Real city map represented by GIS data (for the purpose of this paper using Jiading real data information)Applicability to other citiesOnly cities with one centre and concentric spatial divisionAll types of cities or user-defined regionsNumber of locational factors8 factors for firms and 6 factors for workers17 factors for firms and 13 factors for workersApplicability to measuring policy impacts1. Can only be treated as a reference for the formulation of general policies2. General policies might be applicable to other cities at the macro-level1. Can be used to formulate spatially referenced policies with precise geographical coordinates2. Customised to a specific case study in order to generate policies to compare and contrast across different counties—as each country will have its one creative industries classifications and policiesSpatial allocation of supportive policies from urban governmentOnly five options (Central Business District (CBD), inner urban area, outer urban area, inner suburb, outer suburb)The number of options varies according to the number of administrative divisions of the city being studiedThis paper details this new phase in the development of the CID-USST model. The version of the model presented is named “CID-USST-GIS”.
This model addresses the needs discussed in the preceding paragraphs and in addition features (1) greater flexibility for model users to specify the importance factors that influence office location preferences and workers’ housing location preferences, in addition to possible policies according to findings from a questionnaire conducted in the city under study, and (2) GIS datasets to represent the spatial environment of the new model merged into one layer where the dynamics happen. These features make it possible for policy makers to identify declining/flourishing areas in the city—by referring to simulation results, to “hot spots” produced by the model during its multiple runs, to optimum land use arrangements, and by comparing and contrasting the results of applying different global and/or local policies.Following this introduction, the paper proceeds by briefly describing the CID-USST model (more details of the model can be found in Liu and Silva, ).
Organisation and processing of GIS data for integration with the model in the NetLogo environment is then discussed. The third section then describes an application of the model to the case of Jiading, focusing on an exploration of the dynamics of land use change and the implication for land use planning and policy evaluation and/or development.
The paper then ends with some concluding remarks that highlight the significance of more comprehensive integration of geographical information system (GIS) and agent-based modelling (ABM). 2 Incorporating GIS data with the CID-USST model. 2.1 Introduction to the CID-USST model 2.1.1 The dynamics frameworkThe original CID-USST model was introduced by Liu and Silva (, ). This model was developed in the context of a market economy but under the condition that urban land is owned by the state, and the government has strong administrative power on urban land use arrangement.
Three agent classes were considered in the initial model: creative firms, creative workers and the urban government. Creative firms in the model belong to two sub-sectors: software design and animation design.
Creative workers are the labour force engaged in these two fields. Urban government represents the policy-making institutions of the city under study (in this model, their influence is agents that consist of policies packages). The urban land, the environment in which all the agents’ spatial activities are projected to, is the medium through which the three agent classes are connected. Each urban land plot has a range of properties (such as land use type allowed to be developed, designated plot ratio, terrain, transport availability, building quality) which can change in response to the spatial movements of firms, the workers and the spatial allocations of the urban governmental policy packages. Changes in plot properties mirror the dynamics of urban land use and, in turn, influence the spatial behaviours of the three agent classes.The urban government is responsible for decisions on urban land use that affect creative industries; it influences the system through: (1) urban land use planning whereby overall urban land use arrangements are defined, and (2) supportive policies. Generally, land use planning includes the definition of development zones (such as farm land, green belt), the identification of sites to be regenerated (for housing, office or mix use), the definition of maximum density for each plot, expropriation of land for new development (for housing, office or mix use) and reallocation or compensation to citizens involved.
Supportive policies, on the other hand, are applied as leverage to develop certain urban areas. In other words, only firms on certain land plots designated by the government can benefit from these supportive policies. In the model, three types of supportive policies are considered: (1) tax reduction; (2) lower land/office rent; and (3) trade/milieu promotion (Liu and Silva ).As creative firms need a workforce to generate profits, and creative workers need to earn a living, the spatial movements of these two agent classes are mutually dependent.
However, it cannot be assumed that the workers follow spatially the firms or vice versa, complex recursive interactions between the location of firms and the movement of labour must be considered. Therefore, the model is defined in such a way so that: (1) each firm must try its best to find office space while attracting workers as appropriate, depending on its development status (if a firm cannot find a suitable office within a certain time or fails to employ workers as required, then this firm will fail and will be excluded from the system), and (2) each worker has to find an agreeable residential location while at the same time hunting for a job to support living costs (housing costs).
If this worker fails in either of these two aspects within a tolerable time (defined by the user, i.e. Using questionnaire results), the agent too will be excluded from the process (i.e. It is assumed that the worker abandons the labour market or changes to a different industry).
These processes are termed “agent death” (exclusion from the dynamic system) and agent birth (entry of new creative workers into the dynamic system). Agent birth is moderated by two conditions: (1) the employment rate within the creative industries is sufficiently high to attract the new entrants to the labour market, and (2) the product/service supply is lower than the market demand which can trigger the emergence of creative firms.
2.1.2 The condition-action rules.Urban government (policy packages)The urban/district government exerts its influence upon the dynamic process through land use plans and policy packages (agents in the model). Four parameters are applied in the model to describe the government’s policy strategies: (1) number of policy packages; (2) policy packages’ average duration; (3) priority area to be developed/supported; and (4) business income tax rate for the creative firms. Each policy package is a combination of the three possible policies (see Sect. For details). If the user of the model sets the number of policy packages to “ n”, then the government will formulate “ n” packages and allocate more than half of them to the “prior area”. Each policy package has a duration, and the mean of these “ n” policy packages is the value of “average duration”.
During the simulation period, the government taxes each firm by referring to the “business income tax rate”. Once a policy package’s duration is due, it is withdrawn immediately, redesigned and allocated to another plot of land according to the “prior area”. Generally, not all urban land plots can be used for industries/businesses. For instance, some need to be used as public spaces, while others are conservation/protected areas.
Consequently, the government in the model only allows development on plots granted with development permit. During model run, the user can act as the government and initiate “land expropriation” and “urban regeneration” by pressing “plotrenew” and “newsubflat”. “Land expropriation” happens in protected areas such as green belts and farmlands, while “urban regeneration” always takes place on plots with low building quality. The number of layers and geographical features in each layer depends on the location factors that define the creative firms’ office location preferences and the creative workers’ housing location preferences.
The initial model required eight factors for the firms and five factors for the workers; these factors were dependent on the research done in these two sub-sectors, software design and cartoon design, primarily promoted in Nanjing’s government policy. However, the location decisions of both the creative firms and the creative workers vary across different cities and differ in different sub-sectors. By referring to the literature on this issue (Dainov and Sauka; Hutton; Musterd; Musterd and Gritsai; Vang; Žaucer et al. ), the improved model expands this to consider 17 factors for the firms and 13 factors for the workers (see Table ). Thus, the location utility function for the firms can be rewritten in the form of Eq. ( ) and for the workers in the form of Eq. ( ). Under this framework, the standardised GIS dataset requires 25 layers, each with one relevant geographical factor. The distribution of these geographical features then shapes the heterogeneous landscape of the location marks which are used in the location utility calculation (Table ).
In addition to the GIS dataset, two additional datasets are obtained through questionnaires and/or semi-structured interviews. The first of these datasets is the distribution of the weights on all the location factors (17 factors for the firms and 13 factors for the workers), which serves as the reference for defining the location preference properties of the firms and the workers in the dynamics (Table ), while the second concerns the distribution of policies for firms and workers, i.e. Each possible supportive policy’s number percentage of all the supportive policies (Table ). NetLogo’s “GIS extension” has a built-in primitive (“gis:apply-coverage”) to transfer the polygon’s properties to the “patches”. Plate (1) in Fig.
Illustrates the rules for this procedure, which involves finding all polygons for each intersecting patches. In addition, before the data transfer, two values related to the percentage of a patch being covered by polygons need to be defined: the minimum threshold value (by default 10%) and the maximum threshold value (by default 33%). If the total percentage of the covered area is less than the minimum threshold of a patch being covered by a polygon (patch a in plate (1) of Fig.
), then this patch will be regarded as not covered by any polygons and no property transfer will be executed. If there is only one polygon covering a percentage of area greater than the maximum threshold of a patch being covered b a polygon (patch c in plate (1) of Fig. ), inherit this polygon’s properties (non-numeric properties, such as land use type). However, if it occupies more than one polygon taking up a percentage of area greater than the maximum threshold (patch b in plate (1) of Fig.
), as illustrated by patch b, it will copy the first polygon’s properties (polygon 1 in plate (1) of Fig. ) rather than the others’. Fig. 1Rules for topology operation and data transferRegarding point and polyline data, no primitives are available in NetLogo for property transfer. To deal with point data, each patch in NegLogo is asked to check whether points are contained. If there is more than one point within it, then only the first one’s properties will be copied to the patch.
If there is only one point, then the patch will directly inherit the properties of this point (point 1 in plate (2) of Fig. If the point is located exactly on the bordering line of two patches (point 2 in plate (2) of Fig.
), then one of these two patches will be randomly selected to represent the properties of this point. Similarly, if the point happens to be at the vertex of four patches (point 3 in plate (2) of Fig. ), then one out of the four will be randomly selected as the one inheriting this point’s properties.Regarding polyline data, the model first checks for each patch whether it intersects with the polyline (the patch and the polyline share at least one point). All patches intersecting with the polyline will be treated as a representation of one segment of the polyline. However, if a segment is precisely on the border of two neighbouring patches (segment 1 in plate (3) of Fig. ), then only one of them will be chosen by random to denote this segment.
If there are more than one polylines going through the same patch, then this patch will have the properties of the first polyline but not the others.In practice, not all 25 layers of data will necessarily be available. To accommodate a situation like this, the model allows some of them to be empty by checking the number of features in each of them before data transfer.
If there is no feature in a layer (which means data of this layer is not available), the feature number-checking module will report 0, telling the data-transfer module not to execute transferring so as to avoid computation error. When all the GIS data are incorporated, it is then possible to develop the model in a pure agent-based modelling environment. In other words, this GIS data processing part is a detachable module, which can be coupled with the “set-up” module in the original CID-USST to get data ready in different cases for the execution of the simulation process (the “go” modules). Three matrices are also applied to store the data of the firms’ office location preference, the workers’ housing location preference and the distribution of policies in order to produce the new model that incorporates the GIS data.
As a consequence, the new adapted model reflects this change and is named CID-USST-GIS model.With this new model, it is possible to apply it to other case studies (cities or urban districts) by the expedient of preparing and inputting relevant data according to the standardised datasets. This can be done in two phases: the first one is to define the weight distributions of the factors for firms and workers, as well as the policies (which require minor manual modifications of the code). The second phase is to update the GIS data by saving the 25-layer dataset of the case study in the map folder attached to the code file (.nlogo file).
However, as the number of the sub-districts in different cases varies, the module for drawing the graphs of the spatial distribution of the firms and the workers needs minor revision by referring to the names of the sub-districts. Figure illustrates the user interface of the CID-USST-GIS model. Compared to the original version (CID-USST model) (Liu and Silva ), this model adds a new section (box B in Fig. ) dedicated to policy data. In addition, since the priority areas that the urban government will support depends on the individual case study (i.e. Some areas of the district can be subject to a specific policy and other areas no), a drop-down list of the sub-districts (rather than the five sub-areas as mentioned in Sect. ) is provided accordingly (box A in Fig.
The new version also included additional six buttons (box C in Fig. ) that improve the readability of the outputs in the “world” window by enabling the user to choose a certain theme map (such as distribution of the firms or land use plan map). The Jiading district is located in the northwest of Shanghai (Fig. Under the pressure of economic restructuring, in 2012, Jiading initiated the “Great Incubator” strategy, expecting to attract, cultivate and sustain entrepreneurs and creative workers engaged in creative industries and high-tech industries. One challenge arising from this ambition was how to efficiently and adaptively arrange urban land use for these new industries. The CID-USST-GIS model, as described in the previous sections of this paper, is applied to Jiading as an attempt to support decision-making on this issue. By referring to the standardised GIS dataset (Table ), in the case of Jiading, 17 layers of geographical features (Fig.
) were prepared. Airport data were not included as Jiading does not have its own airport but shares two airports (Pudong International Airport and Hongqiao Airport) with Shanghai municipality. In addition, (water) ports are not considered as there is no port managed by Jiading. The datasets for Internet coverage, primary schools and bus stops are not available, and as a consequence, they are not included in the GIS dataset. So, no property data transfer is executed for these three layers, meaning that each plot’s location mark in each of these three aspects is 0 (because there is no accessibility to the three features).
Thus, the location utility (location mark × weight) derived from these three is 0. The layer of shopping malls is not directly presented as its data were not available. To circumvent this, town centres data were used to represent the convenience for daily shopping. The land use map is a version of the urban land use plan of Jiading (2020). It includes seven land use types: commercial, public services, green space, industrial, residential, R&D and municipal utility (Fig. Green space is strictly protected. In the model, these plots cannot be occupied by creative firms or workers unless the land use type is changed to industrial or residential by Jiading district government.
The firms and workers cannot locate on land plots planned for public facilities and administrative institutions either. As per land use regulations, firms can only occupy plots with land use types of commercial (considering mix land use), R&D and industrial. Workers, on the other hand, can only locate in residential plots. Fig. 5Data of urban land use plan (2020)For the sake of conciseness, only some key results of the case study questionnaire and semi-structured interviews are presented while introducing the model results appear in column 2 and column 4 of Table and Table —weight of each factor for the firms/workers in the case of Jiading (%).Table presents the importance of each of the 17 factors for the creative firms and the 13 factors for the creative workers in Jiading. As seen there, the results indicate that policies (F (14)) play an essential role in shaping the location preferences of creative firms.
In addition, public transport services (F (2) and F (11)) conspicuously outperform the influence of cultural/leisure facilities, the closeness to universities and the proximity to similar industries, factors usually emphasised by scholars (Florida; Glaeser et al. ) as “soft” conditions. The factors that define the creative workers’ location decisions show a very different landscape. The factors that matter most are related to convenience in daily life, such as public transport services (W (6)), closeness to working places (W (5)) and shopping services (W (2)). Lower housing rent is influential but not the most important one.
Factors such as cultural/leisure facilities (W (7)) and good physical environment (W (8)) are just as important as the good accessibility of children’s schooling (W (4)).The importance of the 10 possible policies is depicted in Table. The three mostly valued factors by firms are lower office rent (PF (1)), funding support (PF (2)) and tax reduction (PF (3)). In this case study, as there are no observed policies directly targeted at creative workers, the distribution of the importance of policies for the workers is not considered (i.e. Is left blank).
Netlogo Links Tutorial
3.2 Policy implications of the dynamics of the creative firms. Figure depicts the dynamics of the spatial distribution of creative firms within two five-year plan periods, from 2013 to 2023.
In the first five-year plan period, the 12 areas can be classified into three groups according to their attractiveness to creative firms. The first group is “Industrial park” having the largest number of firms. The middle group includes “Anting”, “Malu” and “Xuxing”.
Netlogo And Qgis
However, being attractive at the beginning does not necessarily mean that this advantage will prevail. For instance, the number of firms in “Xuxing” and “Malu” declines, whereas “Anting” experiences a steady increase, which results in the two-group pattern: “industrial park” and “Anting” rank first and the rest the second. In addition, it can also be seen that the areas being regarded as unattractive by firms early on can over time effectively compete for attracting creative firms as the case of “Anting” illustrates. The simulation results are useful to identify the sub-districts experiencing decline and can help in the policy-making practice to formulate new strategies to restore their attractiveness. In practice, attracting, sustaining and cultivating creative industries require significant investment from the urban government and other potential stakeholders (Comunian ). However, the question is how to decide where and when to put the money and resources?
One response is to invest in the land plots designed for “industrial” or “commercial” activities (brown or red patches in Fig. ) so as to direct creative firms to these areas. This solution is acceptable but still has the problem of not pointing out the priority areas and the timetable for the investment. This problem can be solved by interpreting the outputs of the CID-USST-GIS model. By checking the spatial distribution of firms against the land use plan in three periods (Fig. ), three suggestions can be proposed.
It would be a waste to invest in all land plots; as seen in Fig., some of the areas do not seem to attract firms when the model is running; therefore, it would be better to focus on some of the more dynamic areas that attract more firms and this also means that once we know which areas to prioritise inside each area, the priority should be to focus on the most attractive and dynamic land plots for “ industrial” or “commercial” land as they have the maximum probability of attracting creative industries. The timetable and priority areas for investment are the following: at the starting stage, the focus should be on the “industrial” land plots in “Xuxing”, “commercial” and “R&D” plots in “Malu” (circled areas in 2013 shown in Fig. ); at stage two, there is a need to keep focusing on the previous three locations, while at the same time add another two sites, one in “Anting” and the other in “Waigang” (circled areas in 2018 shown in Fig. ), and the final stage shows a kind of decentralisation which means that there is a need to focus more on the outer areas (the two circled areas in 2023 shown in Fig. ), while at the same time prepare strategies to revitalise the declining areas.
As development and change is a continuous evolutionary process, the land use plans might need alteration and revision in practice to adapt and optimise the profit from land exploitation. For instance, Xuxing (the lake blue line in Fig. ) experiences boom at the early stage (2013–2014), which increases demand for service facilities (food, shopping, etc.) and thus land for service industries. However, after approximately 3 years, this area declines suddenly and keeps a lower growth level (until 2023). This development pattern means that from 2017 onwards, urban regeneration/land use transformation is necessary if the same growth level is to be sustained, which in turn requires revisions of the original land use plan. By referring to the spatial distribution of the workers, all the sub-districts can be categorised into three groups in both the first and the second five-year period: “Malu” and “Xuxing” as the first group; “Huating”, “Zhenxing” and “Waigang” as the last and the rest as the second. However, the areas in each group experience change from period one to two: “Jiangqiao” rises from group two to group one and “Waigang” goes from tier one up to the second tier.
In addition, it can be observed that the creative workers’ spatial distribution pattern has more fluctuations (Fig. ) than creative firms, thus indicating a fierce competition in the labour and housing markets. Workers who cannot get sufficient pay or afford the rising housing prices have to move frequently, or try to look for better-paid jobs. Although every sub-district experiences fluctuation, almost all of them show an overall trend of increase in the number of creative workers. That is, the housing demand increases in every sub-district. This simulation result, however, does not necessarily indicate that the government can distribute investment in all the supposed residential areas equally at the same time which would lead to vast waste of resources.
Netlogo Chooser
So, by comparing the land use plan and the development of the creative workers’ spatial distributions, the policy makers can see that at the beginning, the focus needs to be at the central and southern areas (circled areas in 2013 shown in Fig. But gradually, the focus needs to be shifted to the western parts (circled areas in 2018 shown in Fig. ) and finally to the northern areas and southern sites (circled areas in 2023 shown in Fig. The last two decades have witnessed the emergence and global flourishing of creative industries. It is commonly stated that creative industries tend to be a central goal for cities and regions expecting to generate growth and innovation. Thus, extensive research work has been dedicated to the discussion on creative industries’ contribution to urban economic growth, urban innovation and urban regeneration.
These streams of research, however, have rarely explored the spatiality of creative industries in a comprehensive manner. Even though work has been done on creative industries’ location behaviours and its influence upon the urban built environment, the dynamics of the interaction between creative industries’ development and urban land use has been for the most part ignored. However, the inherent organisational flexibility and spatial adaptability of creative industries present urban policy makers with important challenges that require continuous study so that policy formulation and land use arrangement can be customised and adjusted in an adaptive way.An effort in this direction has been made by Liu and Silva (, ) by developing the CID-USST model.
Initially, the spatial environment of this model was a homogenous and abstract monocentric urban space, and the factors impacting the location preference of the creative firms and the creative workers were fixed. The manipulation of these initial aspects reduced the complexity of the model and the difficulty in the interpretation of the simulated results. While it was an important first phase in this model’s development, the simplification reduced the operability and utility of the system and weakened the policy conclusions that could be gleaned from its use. Moreover, the application of this model to cities with more than one commercial centre presented the risk of misleading conclusions.The new CID-USST-GIS model presented in this paper is capable of processing GIS data and allows model users to adjust the array of locational factors according to the city/region under study.
This flexibility makes it easy to customise the model to different cities and generate specific spatially referenced planning policies. Nevertheless, it increases the complexity of the model and, therefore, increases the challenges in the sensitivity analysis and the results interpretation.